Unlocking Multi-modal Transport using Big Data: India’s Eastern Corridor
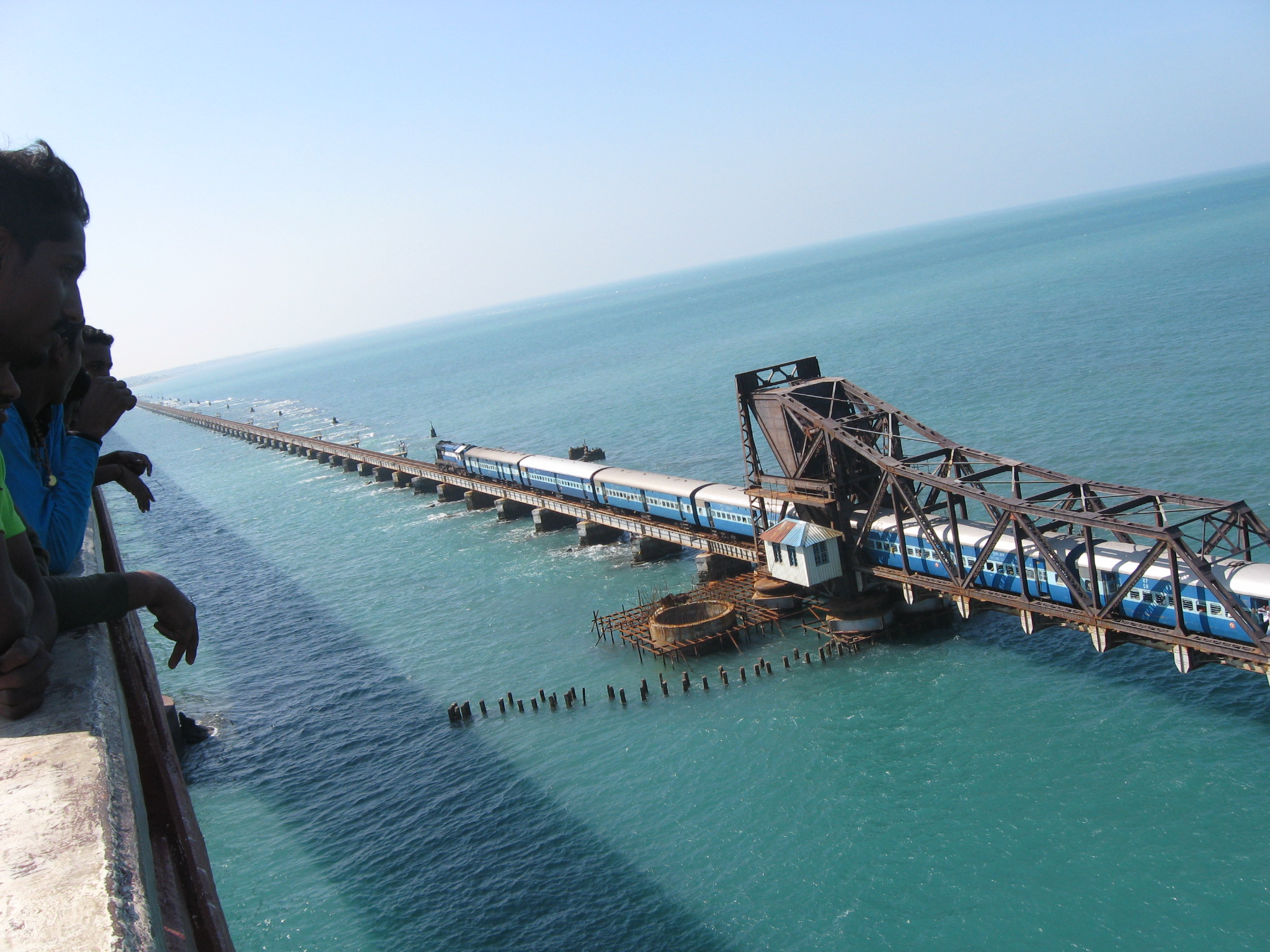
Rail and waterways are the most environmentally friendly modes of transport. They also provide the backbone for accelerated economic growth since they are able to carry huge amounts of freight over longer distances and in an economically efficient manner.
The main handicap for rail and waterways, however, is that these modes of transport must be linked to roads to provide final connectivity to ports, industries or ultimate customers. Therefore in order to leverage large infrastructure investments, it is necessary to have strategically placed transshipment hubs or multimodal stations that allow for multimodal logistics – combining rail, water and road.
Such facilities concentrate critical logistics operations, such as transfer of cargo between modes of transportation, preliminary processing and packaging, consolidation of cargo, and freight storage, all in a single location, thereby taking advantage of economies of scale. However, how are we to efficiently determine the strategic location of these exchanges?

These locations are often chosen based on availability of land or at locations that may be advantageous for a particular mode. In reality, the ideal solution requires optimization of multiple criteria. And here, big data can become a great ally in tackling this challenge.
In this context, the World Bank in India is developing an objective approach for optimizing multi-modal transport on the Eastern Corridor (covering the Indian states of West Bengal, Bihar, Jharkhand, Uttar Pradesh, Haryana and Punjab). There are several on-going investments in transport infrastructure along the Eastern Corridor including the Eastern Dedicated Freight rail project from Ludhiana to Kolkata, which is part of the Golden Quadrilateral of rail corridors connecting the main centers of economic activity. The World Bank also has provided the Government of India with a $2.7 billion loan to build 1,176 kilometers of dedicated freight-only rail lines. The National Waterway 1 system is also an opportunity to use waterways for freight on the River Ganges between Haldia to Allahabad. The waterways and railways are close to several state and national highways that together could form a network of transport infrastructure that provides a multi-modal backbone for greater economic growth in the region.
The advantage for India – from a national policy perspective – is that a critical transport and logistics network is still to be built, and so the country can pursue an optimal multimodal transport strategy that maximizes cost efficiency, responds to needs of customers and reduces losses for users while reducing the impact of freight transport on the environment. Ordinary people, for example, will benefit from reduced congestion (see story related) in cities as more freight is transported on rail and waterways, and capacity is released from existing rail lines, which currently have to carry both freight and passengers. This will also lead to communities along the corridor having better access to employment opportunities, health, education and other services.
To encourage multiple modes, however, it is crucial to find the optimal location to provide freight exchanges between modes. In the approach developed through the Eastern Corridor project, we used spatial information to develop and test an optimization model that would find the best location for such an exchange hub. Since we wanted the problem to be solvable, we developed and tested a pilot optimization model using only open data and open software. To find the optimal location of the hub, we used a combination of project specific data, including GIS data on the location of the existing/planned infrastructure.
The optimization model looks to minimize the total logistics cost of transporting goods, in terms of infrastructure travel cost and travel time of goods, while taking into consideration local issues. The optimization model utilizes primarily geo-spatial information to find the distance information in the area. This is then converted into multiple matrices that are utilized by the linear optimization model.
To test the validity and applicability of the model, we created and run a pilot over a 175km by 40km stretch in the corridor. To reduce the complexity of the model, we applied a 5km by 5km grid over the study area, where every grid cell represented a possible location for multimodal port. This essentially converts a continuous model (which will probably be unsolvable due to the study area) into a discrete model.

For the pilot study, we relied on open data sources, including:
- three different networks: rail, road, and water;
- commodity flows, as represented by nighttime lights (VIIR, NOAA November 2014);
- location-based variables: urban extents (GRUMP 2000), population (WorldPop), land cover; and
- cost information for nine different commodities.
We have tested out this model in a pilot area where we identified the location of a multimodal port that minimized exchange costs for nine basic commodities. The image above shows the optimal location of our pilot model. This location produces a logistics cost savings of 2-5% comparing to other locations that might have been chosen simply by looking at the availability of land. Given the successful pilot of the basic model, we will now expand the research to include the entire eastern corridor.
The availability of open spatial information has dramatically improved our ability to plan efficient transportation systems that take advantage of economies of scale through intermodal ports. And we were able to test out an entire pilot study using only open data. Big data allows us to understand networks and multimodality, and to optimize our transportation systems. Utilizing these techniques, India can build its freight infrastructure in an integrated network and across modes, as well as prioritizing high-return infrastructure programs.
We think other countries could also benefit from this experience. What other possibilities do you see for unlocking multimodal transport through big data?